Understanding Image Segmentation Labeling Tools: Elevate Your Data Annotation Strategy
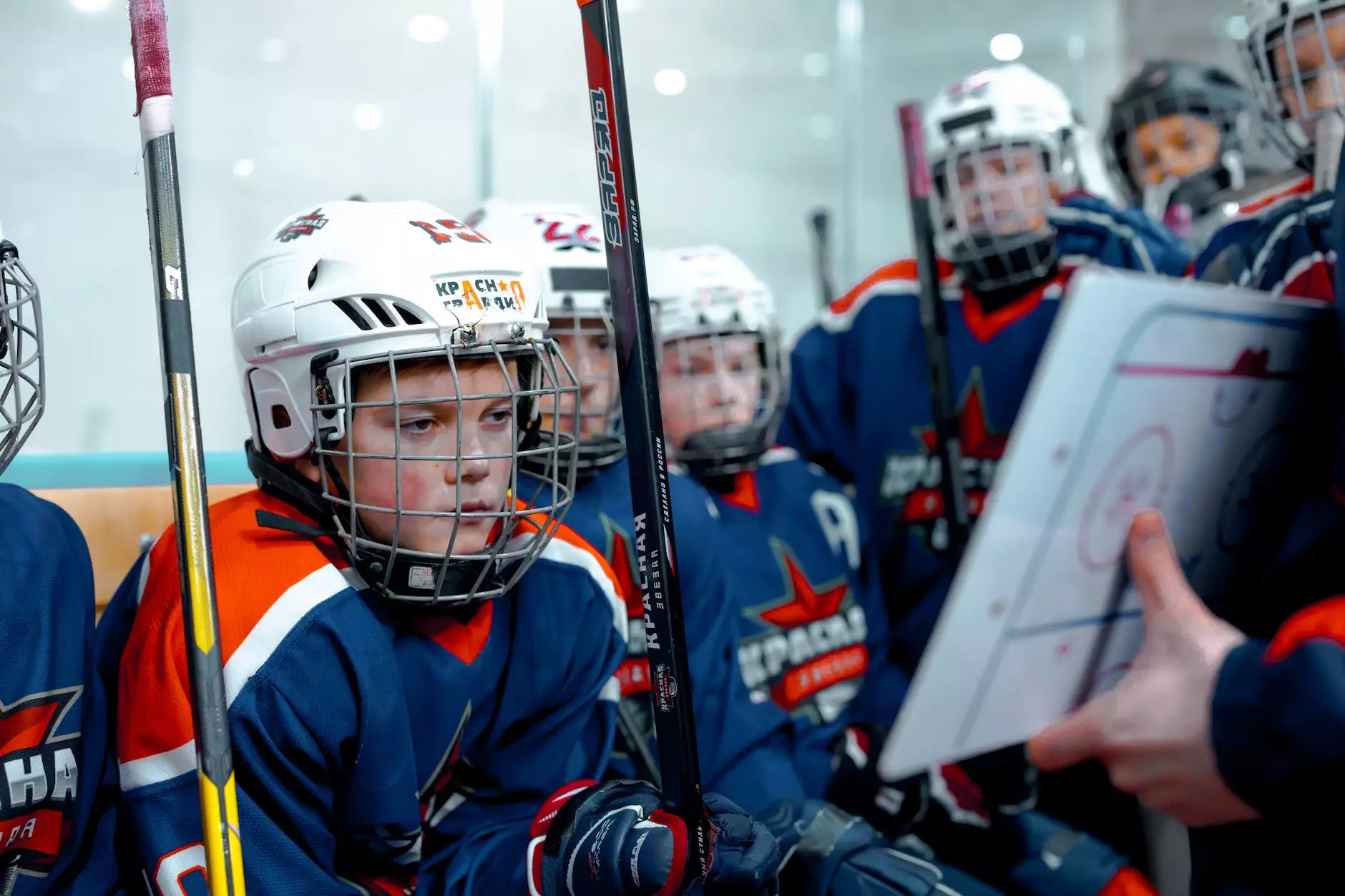
In the ever-evolving landscape of artificial intelligence and machine learning, the demand for accurate and efficient data annotation has never been more critical. Image segmentation labeling tools play an essential role in enabling computer vision applications by accurately identifying and labeling individual areas within images. By using such tools effectively, businesses can vastly improve their AI model training processes and significantly enhance the quality of their outcomes.
What is Image Segmentation?
Image segmentation is a computer vision technique that involves partitioning an image into multiple segments or regions, making it easier to analyze the image's contents. Each segment typically corresponds to a distinct object or part of an object, allowing algorithms to understand and process each component separately. This technique is pivotal in various fields, including autonomous driving, medical image analysis, and augmented reality.
The Importance of Image Segmentation in AI
The significance of accurate image segmentation cannot be overstated. It directly impacts the performance of machine learning algorithms, affecting how well they can identify, classify, and predict outcomes based on visual data. Some of the key advantages include:
- Improved Model Accuracy: Precise segmentation results equate to better predictions and classifications in AI applications.
- Enhanced Data Utilization: Properly labeled datasets maximize the effectiveness of training models, ensuring that they learn from accurate representations of data.
- Time Efficiency: Automated image segmentation labeling tools can significantly reduce the time required for manual annotation, speeding up the overall project timeline.
- Better Quality Control: Using advanced image segmentation tools helps in maintaining consistency and accuracy across large datasets.
Features of a High-Quality Image Segmentation Labeling Tool
When searching for an image segmentation labeling tool, it's crucial to assess its features and capabilities. A top-tier tool will typically include:
1. User-Friendly Interface
A clean, intuitive interface allows users to navigate the tool easily, making the annotation process more efficient.
2. Automated Segmentation
Advanced algorithms that can automatically segment images reduce manual effort and improve turnaround time.
3. High Customizability
The ability to customize segmentation categories and label styles allows for a tailored approach to meet specific project requirements.
4. Quality Assurance Features
In-built mechanisms for error detection and an option for review ensure high-quality outputs.
5. Integration Capabilities
Seamless integration with other AI tools and platforms enhances workflow efficiency, allowing teams to utilize the tool in their existing environments.
Utilizing Keylabs.ai for Image Segmentation
Keylabs.ai stands out in the crowded field of data annotation tools with its state-of-the-art image segmentation capabilities. Here’s how our platform enhances your annotation processes:
1. Comprehensive Data Annotation Platform
Our platform supports a range of annotation types, including bounding boxes, keypoints, and most importantly, image segmentation, providing versatility for diverse projects.
2. Machine Learning-Assisted Annotation
By leveraging machine learning, Keylabs.ai can expedite the segmentation process, generating high-quality labeled data in a fraction of the time compared to traditional methods.
3. Extensive Collaboration Tools
Our platform promotes team collaboration with features that enable real-time sharing of annotations, enhancing productivity and minimizing errors.
4. Robust Customer Support
We pride ourselves on offering exceptional customer support, ensuring that our users can maximize the utility of the image segmentation labeling tools available on our platform.
Real-World Applications of Image Segmentation
The applications of image segmentation are vast and varied, impacting numerous industries. Here are a few notable instances:
1. Autonomous Vehicles
In self-driving car technology, image segmentation helps in identifying different street components, such as pedestrians, vehicles, and road signs, thereby ensuring safer navigation.
2. Medical Imaging
Medical professionals utilize image segmentation to analyze scan images, aiding in accurate diagnosis and treatment planning, such as tumor detection in MRI scans.
3. Augmented Reality (AR)
In AR applications, effective segmentation allows for the overlay of digital content onto the real-world view, creating immersive experiences for users.
4. Facial Recognition
Image segmentation plays a crucial role in facial recognition technology, enabling software to differentiate facial features for various security and user experience applications.
Challenges in Image Segmentation
Despite its advantages, image segmentation comes with its own set of challenges:
1. Complex Data Requirements
Generating high-quality segmentations requires substantial amounts of accurately labeled data, which can be time-consuming and costly to produce.
2. Variability in Image Quality
Images captured under different conditions may exhibit variations that complicate segmentation, necessitating robust algorithms capable of handling diverse inputs.
3. Subjectivity in Labeling
Different annotators may have varying interpretations of segment boundaries, leading to inconsistencies unless stringent quality control measures are in place.
The Future of Image Segmentation and Annotation Tools
The future of image segmentation labeling tools looks promising, with advancements in AI and machine learning driving innovation. We can expect:
1. Increased Automation
As algorithms evolve, the automation of image segmentation will become more sophisticated, allowing for faster and more accurate labeling.
2. Better Integration with AI Solutions
Future tools will seamlessly integrate with existing AI and machine learning platforms, making it easier for businesses to adopt and implement these technologies.
3. Enhanced User Experience
With ongoing developments in user interface design, tools will become even more accessible and easy to use, opening the door for users from non-technical backgrounds.
4. Expanded Applications
Innovations in image segmentation will lead to new applications and industries adopting these technologies, further extending their impact on business and society.
Conclusion
In conclusion, image segmentation labeling tools are pivotal in harnessing the power of visual data for AI applications. Platforms like Keylabs.ai provide comprehensive solutions that streamline the annotation process, ensuring high-quality outputs critical for model training. As technology advances, embracing these tools will not only enhance the accuracy of AI systems but also drive innovation across various sectors. Investing in the right image segmentation tools today positions your business for a successful, data-driven future.
For more information and to explore our offerings, visit us at Keylabs.ai.